Checking for non-preferred file/folder path names (may take a long time depending on the number of files/folders) ...
This resource contains some files/folders that have non-preferred characters in their name. Show non-conforming files/folders.
This resource contains content types with files that need to be updated to match with metadata changes. Show content type files that need updating.
Data from Harmon et al. (2021), Exploring environmental factors that drive diel variations in tree water storage using wavelet analysis
Authors: |
|
|
---|---|---|
Owners: |
|
This resource does not have an owner who is an active HydroShare user. Contact CUAHSI (help@cuahsi.org) for information on this resource. |
Type: | Resource | |
Storage: | The size of this resource is 18.4 MB | |
Created: | Mar 15, 2021 at 3:21 a.m. | |
Last updated: | Aug 09, 2021 at 3:34 a.m. (Metadata update) | |
Published date: | Aug 09, 2021 at 3:33 a.m. | |
DOI: | 10.4211/hs.6e102de63a7943e1900aa8c6a8d412ac | |
Citation: | See how to cite this resource |
Sharing Status: | Published |
---|---|
Views: | 1577 |
Downloads: | 49 |
+1 Votes: | Be the first one to this. |
Comments: | 1 comment |
Abstract
These data are published in Harmon, R., Barnard, H., Day-Lewis, F.D., Mao, D., and Singha, K. (2021). Exploring environmental factors that drive diel variations in tree water storage using wavelet analysis. Frontiers in Water, doi: 10.3389/frwa.2021.682285.
Internal water storage within trees can be a critical reservoir that helps trees overcome both short- and long-duration environmental stresses. We monitored changes in internal tree water storage in a ponderosa pine using moisture probes, a dendrometer, and time-lapse electrical resistivity imaging (ERI) to investigate how patterns of in-tree water storage are affected by changes in sapflow rates, soil moisture, and meteorologic factors such as vapor pressure deficit. ERI measurements are influenced by changes in moisture, temperature, solute concentration, and material properties; thus, to evaluate changes in moisture based on ERI, the first three factors must be considered. Measurements of xylem fluid electrical conductivity were constant in the early growing season, while inverted sapwood electrical conductivity steadily increased, suggesting that increases in electrical conductivity of the sapwood did not result from an increase xylem fluid electrical conductivity. Seasonal increases in stem electrical conductivity corresponded with seasonal increases in trunk diameter, suggesting that increased electrical conductivity may result from new growth. Changes in diel amplitudes of inverted sapwood electrical conductivity, which correspond to diel changes in sapwood moisture, indicated that tree water storage use was greatest ~4-5 days after storm events, when sapwood inverted electrical conductivity measurements suggest internal stores were high. A decrease in diel amplitudes of inverted sapwood electrical conductivity during dry periods, suggest that the ponderosa pine relied on internal water storage to supplement transpiration demands, but as drought conditions progressed, tree water storage contributions to transpiration decreased. Wavelet analyses indicated that lag times between inverted sapwood electrical conductivity and sapflow increased after storm events, suggesting that as soils dried reliance on internal water storage increased and the time required to refill daily deficits in internal water storage increased. Lag times peaked when soil moisture returned to pre-storm event levels and then decreased as drought progressed. Short time lags between sapflow and inverted sapwood electrical conductivity corresponded with dry conditions, when ponderosa pine are known to reduce stomatal conductance to avoid xylem cavitation. Time-lapse ERI- and wavelet-analysis results highlighted the important role internal tree water storage plays in supporting transpiration throughout the course of a day, and during periods of declining subsurface moisture.
Subject Keywords
Coverage
Spatial
Temporal
Start Date: | |
---|---|
End Date: |
Content
Readme.txt
Overview of information on this Hydroshare page: Sapflow, soil moisture, sapflow, and metrological data were collected between May and November 2018, as described in the Frontiers in Water manuscript "Exploring Environmental Factors That Drive Diel Variations in Tree Water Storage Using Wavelet Analysis". In the Content section here, you will find: 1) The Subsurface Data folder includes: Uncalibrated soil moisture data collected with METER ECH2O EC-5 sensors located one-meter away from the study tree and at 10, 20, and 40-cm depth. 2) The Surface Data folder includes: a. Climate data measured every 10 minutes at the south-facing Gordon Gulch weather station. Data includes precipitation, wind speed, solar radiation, air temperature, and relative humidity (see https://www.hydroshare.org/resource/d66f1f3239a94c7682c71217b1a94e0b/). 2) Tree Measurements folder includes: a. Dendrometer measurements using a METER digital dendrometer (https://www.metergroup.com/environment/#d6), which was attached 1.50-m above the ground surface. b. Sapflow data [cm^3/hr] collected at 1.5m above ground surface on the east and west sides of the study tree (recorded every 30min). c. Cross-sectional temperature profile of the tree trunk. Measurements were collected at 2, 10, and 20 cm depth on the south- and north-facing sides of the tree. Measurements were collected every 30 minutes. d. Sapwood volumetric water content data measured with METER TEROS 12 frequency-domain reflectometry sensor (https://www.metergroup.com/environment/products/teros-12/), which was inserted into the xylem 2m above the ground surface, which provided an indirect measure of VWC and measured temperature every 30min. e. Sapwood Electrical Conductivity: - electrical resistivity imaging data. - bulk electrical conductivity of xylem water measured by coring into the xylem of nearby ponderosa pines. - electrical conductivity data measured with a METER TEROS 12 frequency-domain reflectometry sensor (https://www.metergroup.com/environment/products/teros-12/), which was inserted into the xylem 2m above the ground surface.
Related Resources
This resource is referenced by | Harmon, R., Barnard, H., Day-Lewis, F.D., Mao, D., and Singha, K. (2021). Exploring environmental factors that drive diel variations in tree water storage using wavelet analysis. Frontiers in Water, doi: 10.3389/frwa.2021.682285. |
Credits
Funding Agencies
This resource was created using funding from the following sources:
Agency Name | Award Title | Award Number |
---|---|---|
National Science Foundation | From Roots to Rock - Linking Evapotranspiration and Groundwater Fluxes in the Critical Zone | EAR1446161 and EAR1446231 |
National Science Foundation | Collaborative Research: Network Cluster: Bedrock controls on the deep critical zone, landscapes, and ecosystems | EAR-2012408 |
Contributors
People or Organizations that contributed technically, materially, financially, or provided general support for the creation of the resource's content but are not considered authors.
Name | Organization | Address | Phone | Author Identifiers |
---|---|---|---|---|
Jackie Randell | Colorado School of Mines | |||
Fred Day-Lewis | Pacific Northwest National Lab | |||
Deqiang Mao | Shandong University | |||
Aaron Engers | Colorado School of Mines |
How to Cite
This resource is shared under the Creative Commons Attribution-NoCommercial CC BY-NC.
http://creativecommons.org/licenses/by-nc/4.0/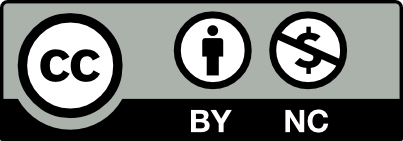
Comments
Kamini Singha 8 months ago
A couple of notes about the published in-tree temperature data here:
ReplyThe spreadsheet here has temperature data labeled for 2, 10, and 20 cm depth. The 20 cm depth label is a typo and should be 22 cm depth. These data are for the probe inserted on the north side of the tree, starting in January of 2018, as outlined in the linked paper, above.
It appears we didn't publish the data from the south side of the tree, which has 26.5, 34, 38, 42, and 46 cm measurements. The south probe did not go in until June of 2018. We apologize for this error, and will share an updated .csv with anyone who would like the file since we cannot change this repository or add an extra file here. Apologies for this oversight.
Lastly, the negative temperatures were collected during the winter, when the sensor didn't collect meaningful data.
New Comment