Machine Learning-Derived Channel Width and Depth for the National Hydrologic Geospatial Fabric in CONUS
Authors: | |
---|---|
Owners: | Arash Modaresi Rad |
Type: | Resource |
Storage: | The size of this resource is 72.7 MB |
Created: | May 10, 2024 at 5:49 p.m. |
Last updated: | Aug 23, 2024 at 12:25 p.m. (Metadata update) |
Published date: | Aug 23, 2024 at 12:24 p.m. |
DOI: | 10.4211/hs.d147fcf554a54b2aaa4f146f85da0e03 |
Citation: | See how to cite this resource |
Content types: | Single File Content |
Sharing Status: | Published |
---|---|
Views: | 820 |
Downloads: | 19 |
+1 Votes: | Be the first one to this. |
Comments: | No comments (yet) |
Abstract
This dataset focuses on reach-averaged estimation of river channel geometry, including top-width and depth, crucial for water flow prediction and flood mapping. Leveraging HYDRoacoustic data from the Surface Water Oceanographic Topography (HYDRoSWOT) program, we develop a machine learning model to predict channel geometry using data from the National Water Model, National Hydrologic Geospatial Fabric network, and other geospatial datasets. Our model demonstrates good fit within the Continental United States, with better performance observed in flatter regions. Covering nearly 2.7 million reaches in the US, this dataset is indexed to the National Hydrologic Geospatial Fabric network. However, in estuaries, particularly near river mouths where it widens into the coastal zone, there are no recorded Acoustic Doppler Current Profiler (ADCP) measurements in HYDRoSWOT, leading to unreliable model accuracy. Additionally, limitations in the training dataset, particularly the primary significant feature of ML models—100% annual exceedance probability discharge derived from the NWM—diminish skill in this exceedance probability, impacting the overall model goodness-of-fit. We provide estimates of channel geometry for two conditions: 100% and 50% annual exceedance probability, based on NWM historical retrospective data..
Subject Keywords
Coverage
Spatial
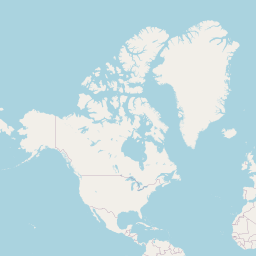

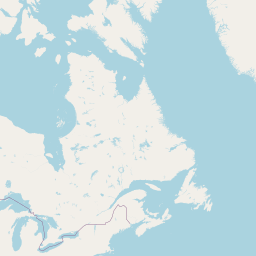
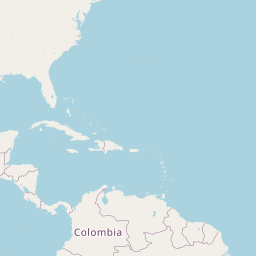
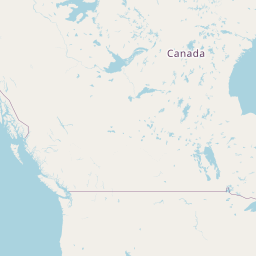

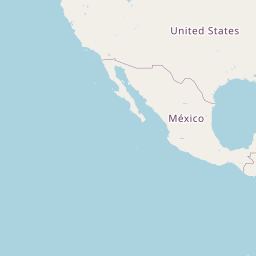

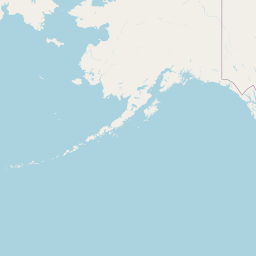
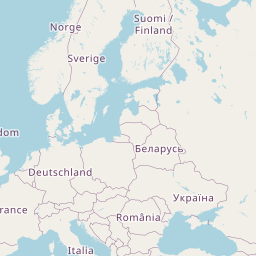
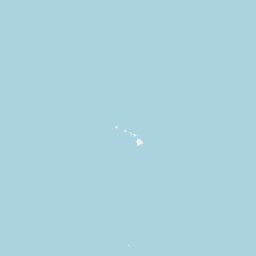
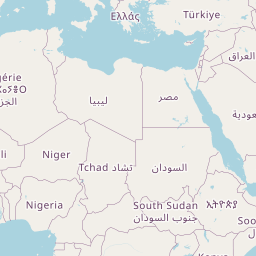
Content
Additional Metadata
How to Cite
This resource is shared under the Creative Commons Attribution CC BY.
http://creativecommons.org/licenses/by/4.0/
Comments
There are currently no comments
New Comment