Incorporating Multi-source Remotely Sensed Vegetation Indices into Hydrologic Simulations in the Upper Colorado River Basin (UCRB)
Authors: | |
---|---|
Owners: | Ruijie ZengQianqiu Longyang |
Type: | Resource |
Storage: | The size of this resource is 2.7 GB |
Created: | Jun 23, 2023 at 6:36 p.m. |
Last updated: | Jun 06, 2024 at 8:27 p.m. |
Citation: | See how to cite this resource |
Content types: | Single File Content |
Sharing Status: | Public |
---|---|
Views: | 976 |
Downloads: | 56 |
+1 Votes: | Be the first one to this. |
Comments: | No comments (yet) |
Abstract
Vegetation plays a crucial role in atmosphere-land water and energy exchanges, global carbon cycle and basin water conservation. Land Surface Models (LSMs) typically represent vegetation characteristics by monthly climatologic index (e.g., green vegetation fraction GVF, leaf area index). However, static vegetation parameterization does not capture dynamic-varying vegetation characteristics, such as responses to climatic fluctuation, long-term trend and interannual variability. This study developed a machine learning accelerated approach to quantify the impacts of dynamic-varying vegetation on the magnitude, seasonality, and biotic and abiotic components of hydrologic fluxes. A deep learning-based surrogate of Noah provided a rapid diagnostic tool to fuse GVF from seven remotely sensed products into LSM. Using the Upper Colorado River Basin (UCRB) as a test case, we found that dynamic-varying vegetation provides more buffering effect to climate fluctuation than the static vegetation configuration, leading to higher total evapotranspiration (thus lower water yield) and smaller evapotranspiration interannual variability. In addition, dynamic-varying vegetation from multi-source remote sensing products consistently predicts larger evaporation abiotic components (e.g., soil evaporation), which are partially compensated by smaller evaporation biotic components (e.g., transpiration). Based on the hydrologic sensitivity analysis to vegetation, we found that vegetation removal in the sparsely vegetated sandy soil regions of the UCRB would lead to the most effective water yield increase. This study highlights the importance of explicit representation of vegetation dynamics in climate change and land management assessment.
Subject Keywords
Coverage
Spatial
Temporal
Start Date: | 10/01/1979 |
---|---|
End Date: | 09/30/2016 |
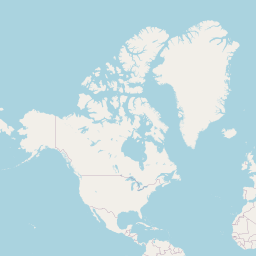
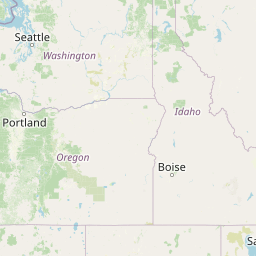
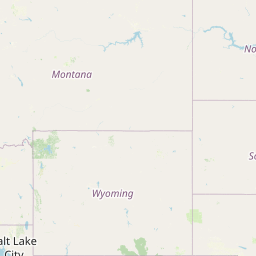

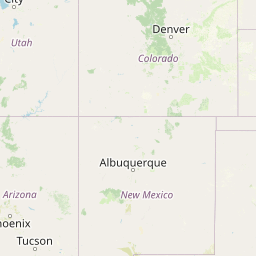
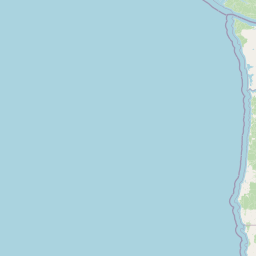
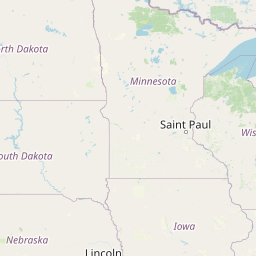
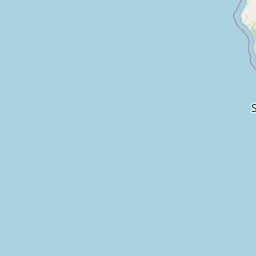
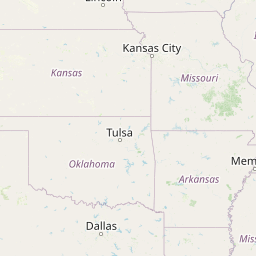
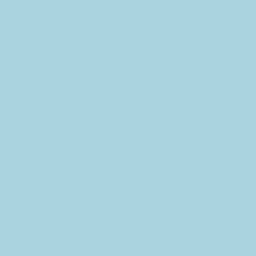
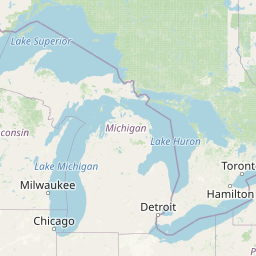
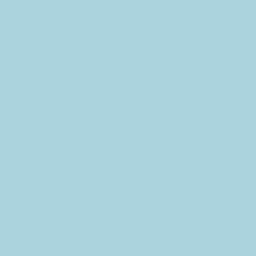
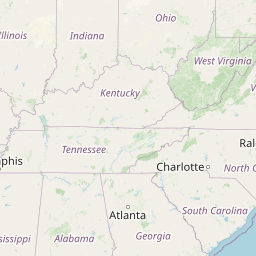
Content
Related Geospatial Features
This HydroShare resource is linked to the following geospatial features
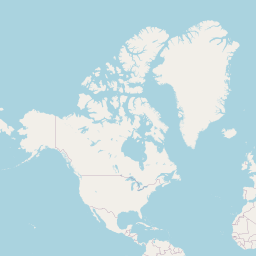

How to Cite
This resource is shared under the Creative Commons Attribution CC BY.
http://creativecommons.org/licenses/by/4.0/
Comments
There are currently no comments
New Comment